Reduce event noise automatically
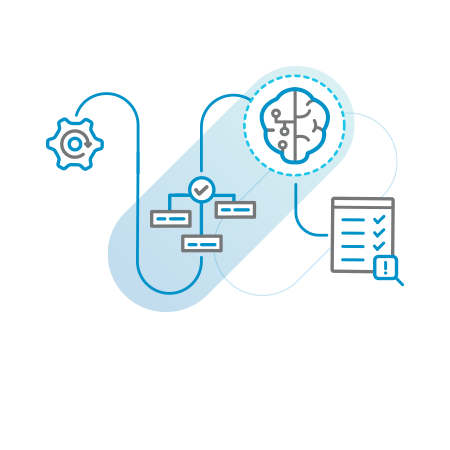
Consolidate data sources from across the enterprise in a unified, actionable view. Use artificial intelligence and machine learning (AI/ML)-driven predictive analytics to:
- Identify operational issues quickly
- Reduce work hours by 20 percent with automated remediations
Featured products
- BMC Helix Operations Management with AIOps: Award-winning service-centric monitoring, advanced event management, and AI/ML-based root cause isolation to reduce MTTR and improve agility
- BMC AMI Ops: AI-powered mainframe observability suite with actionable intelligence, embedded expertise, and simplified management